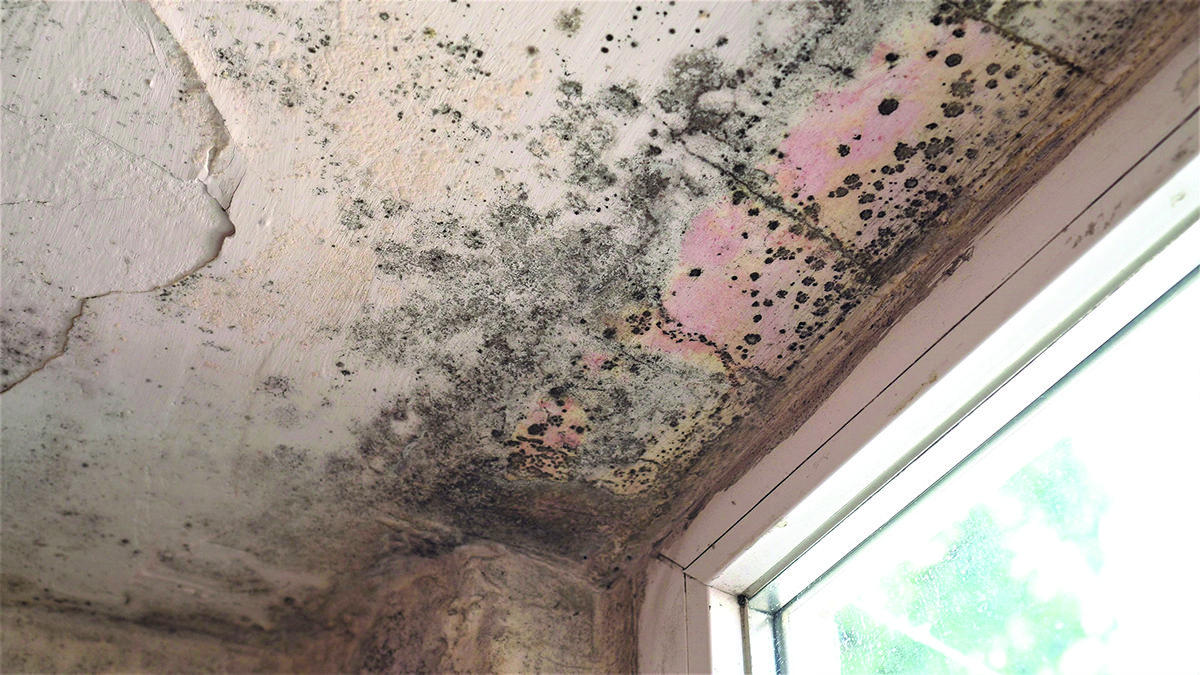
A new project aims to advance understanding of bioaerosol exposure and associated health impacts.
Supported by UKRI AI for Health funding, environmental scientists will bring together internet-of-things (IoT) sensor arrays and AI techniques to measure the pollen and spores within the air we breathe.
Machine learning algorithms will classify the pollen and fungal spore species of interest and generate approaches to detect them in real time – improving current detection techniques which can be slow and expensive.
Working with counterparts at the University of Manchester and UK Health Security Agency (UKHSA), a group of University of Birmingham researchers will assess how efficient the new AI and IoT tools are in meeting the bioaerosol detection and forecasting needs of project partners such as the Met Office and Environment Agency.
Pollen and fungal spores are linked to respiratory illnesses, including some that can be deadly. Project lead Professor Francis Pope, said existing methods of detecting spores can be expensive and time consuming – hugely limiting their use.
The project will see IoT sensors measuring the size distribution of small atmospheric particles present within the air, the sources and composition of which are many and varied.
These particles include bioaerosols composed of fragments from the biosphere, including pollen and fungal spores.
Finding these bioaerosols within much larger populations of other atmospheric aerosols is difficult, but pollen and fungal spores have well-defined sizes so AI approaches could classify species of interest and generate approaches to detect them in real time. This would allow for real-time forecasts of pollen and spore species of interest.
Currently the regulatory detection of pollen and fungal spores is limited because of the high associated costs. For example, the UK Met Office currently only has available 11 regulatory grade sites for pollen monitoring from which the data for their pollen forecast is drawn. Similarly, UK agencies lack cheap methodologies to detect fungal spores in both outdoor and indoor locations.